Inductive Program Synthesis Guided by Observational Program Similarity
Author(s)
Feser, Jack; Dillig, I??l; Solar-Lezama, Armando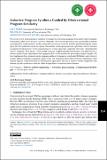
Download3622830.pdf (520.8Kb)
Publisher with Creative Commons License
Publisher with Creative Commons License
Creative Commons Attribution
Terms of use
Metadata
Show full item recordAbstract
We present a new general-purpose synthesis technique for generating programs from input-output examples. Our method, called metric program synthesis, relaxes the observational equivalence idea (used widely in bottom-up enumerative synthesis) into a weaker notion of observational similarity, with the goal of reducing the search space that the synthesizer needs to explore. Our method clusters programs into equivalence classes based on an expert-provided distance metric and constructs a version space that compactly represents “approximately correct” programs. Then, given a “close enough” program sampled from this version space, our approach uses a distance-guided repair algorithm to find a program that exactly matches the given input-output examples. We have implemented our proposed metric program synthesis technique in a tool called SyMetric and evaluate it in three different domains considered in prior work. Our evaluation shows that SyMetric outperforms other domain-agnostic synthesizers that use observational equivalence and that it achieves results competitive with domain-specific synthesizers that are either designed for or trained on those domains.
Date issued
2023-10-16Department
Massachusetts Institute of Technology. Department of Electrical Engineering and Computer ScienceJournal
Proceedings of the ACM on Programming Languages
Publisher
ACM
Citation
Feser, Jack, Dillig, I??l and Solar-Lezama, Armando. 2023. "Inductive Program Synthesis Guided by Observational Program Similarity." Proceedings of the ACM on Programming Languages, 7 (OOPSLA2).
Version: Final published version
ISSN
2475-1421
Collections
The following license files are associated with this item: