Driving and suppressing the human language network using large language models
Author(s)
Tuckute, Greta; Sathe, Aalok; Srikant, Shashank; Taliaferro, Maya; Wang, Mingye; Schrimpf, Martin; Kay, Kendrick; Fedorenko, Evelina; ... Show more Show less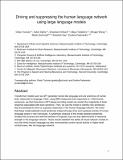
DownloadTuckute_20231031_MS-short-refs_post-man-proofs-clean.pdf (40.95Mb)
Open Access Policy
Open Access Policy
Creative Commons Attribution-Noncommercial-Share Alike
Terms of use
Metadata
Show full item recordAbstract
Transformer models such as GPT generate human-like language and are highly predictive of human brain responses to language. Here, using fMRI-measured brain responses to 1,000 diverse sentences, we first show that a GPT-based encoding model can predict the magnitude of brain response associated with each sentence. Then, we use the model to identify new sentences that are predicted to drive or suppress responses in the human language network. We show that these model-selected novel sentences indeed strongly drive and suppress activity of human language areas in new individuals. A systematic analysis of the model-selected sentences reveals that surprisal and well-formedness of linguistic input are key determinants of response strength in the language network. These results establish the ability of neural network models to not only mimic human language but also noninvasively control neural activity in higher-level cortical areas, like the language network.
Date issued
2024-01-03Department
Massachusetts Institute of Technology. Department of Brain and Cognitive SciencesJournal
Nature Human Behavior
Publisher
Springer Nature
Citation
Tuckute, G., Sathe, A., Srikant, S. et al. Driving and suppressing the human language network using large language models. Nat Hum Behav (2024).
Version: Author's final manuscript
ISSN
2397-3374
Collections
The following license files are associated with this item: