HYPER: Learned Hybrid Trajectory Prediction via Factored Inference and Adaptive Sampling
Author(s)
Huang, Xin; Rosman, Guy; Gilitschenski, Igor; Jasour, Ashkan; McGill, Stephen G.; Leonard, John J.; Williams, Brian C.; ... Show more Show less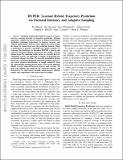
Download2110.02344.pdf (1.764Mb)
Open Access Policy
Open Access Policy
Creative Commons Attribution-Noncommercial-Share Alike
Terms of use
Metadata
Show full item recordAbstract
Modeling multi-modal high-level intent is important for ensuring diversity in trajectory prediction. Existing approaches explore the discrete nature of human intent before predicting continuous trajectories, to improve accuracy and support explainability. However, these approaches often assume the intent to remain fixed over the prediction horizon, which is problematic in practice, especially over longer horizons. To overcome this limitation, we introduce HYPER, a general and expressive hybrid prediction framework that models evolving human intent. By modeling traffic agents as a hybrid discrete-continuous system, our approach is capable of predicting discrete intent changes over time. We learn the probabilistic hybrid model via a maximum likelihood estimation problem and leverage neural proposal distributions to sample adaptively from the exponentially growing discrete space. The overall approach affords a better trade-off between accuracy and coverage. We train and validate our model on the Argoverse dataset, and demonstrate its effectiveness through comprehensive ablation studies and comparisons with state-of-the-art models.
Date issued
2022-05-23Department
Massachusetts Institute of Technology. Computer Science and Artificial Intelligence LaboratoryPublisher
IEEE
Citation
Huang, Xin, Rosman, Guy, Gilitschenski, Igor, Jasour, Ashkan, McGill, Stephen G. et al. 2022. "HYPER: Learned Hybrid Trajectory Prediction via Factored Inference and Adaptive Sampling."
Version: Author's final manuscript