Integrated data-driven modeling and experimental optimization of granular hydrogel matrices
Author(s)
Verheyen, Connor A.; Uzel, Sebastien G.M.; Kurum, Armand; Roche, Ellen T.; Lewis, Jennifer A.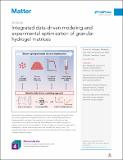
Download1-s2.0-S2590238523000115-main.pdf (4.956Mb)
Publisher with Creative Commons License
Publisher with Creative Commons License
Creative Commons Attribution
Terms of use
Metadata
Show full item recordAbstract
Granular hydrogel matrices have emerged as promising candidates for cell encapsulation, bioprinting, and tissue engineering. However, it remains challenging to design and optimize these materials given their broad compositional and processing parameter space. Here, we combine experimentation and computation to create
granular matrices composed of alginate-based bioblocks with controlled structure, rheological properties, and injectability profiles. A custom machine learning pipeline is applied after each phase of experimentation to automatically map the multidimensional input-output patterns into condensed data-driven models. These models are used to assess generalizable predictability and define high-level design rules to guide subsequent phases of development and characterization. Our integrated, modular approach opens new avenues to understanding and controlling the behavior of complex soft materials.
Date issued
2023-03Department
Harvard University--MIT Division of Health Sciences and Technology; Massachusetts Institute of Technology. Institute for Medical Engineering & Science; Massachusetts Institute of Technology. Department of Mechanical EngineeringJournal
Matter
Publisher
Elsevier BV
Citation
Verheyen, Connor A., Uzel, Sebastien G.M., Kurum, Armand, Roche, Ellen T. and Lewis, Jennifer A. 2023. "Integrated data-driven modeling and experimental optimization of granular hydrogel matrices." Matter, 6 (3).
Version: Final published version
ISSN
2590-2385
Keywords
General Materials Science