A new computational framework for log-concave density estimation
Author(s)
Chen, Wenyu; Mazumder, Rahul; Samworth, Richard J.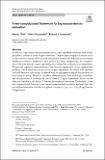
Download12532_2024_Article_252.pdf (4.210Mb)
Publisher with Creative Commons License
Publisher with Creative Commons License
Creative Commons Attribution
Terms of use
Metadata
Show full item recordAbstract
In statistics, log-concave density estimation is a central problem within the field of nonparametric inference under shape constraints. Despite great progress in recent years on the statistical theory of the canonical estimator, namely the log-concave maximum likelihood estimator, adoption of this method has been hampered by the complexities of the non-smooth convex optimization problem that underpins its computation. We provide enhanced understanding of the structural properties of this optimization problem, which motivates the proposal of new algorithms, based on both randomized and Nesterov smoothing, combined with an appropriate integral discretization of increasing accuracy. We prove that these methods enjoy, both with high probability and in expectation, a convergence rate of order 1/T up to logarithmic factors on the objective function scale, where T denotes the number of iterations. The benefits of our new computational framework are demonstrated on both synthetic and real data, and our implementation is available in a github repository LogConcComp (Log-Concave Computation).
Date issued
2024-04-30Department
Massachusetts Institute of Technology. Operations Research Center; Sloan School of ManagementJournal
Mathematical Programming Computation
Publisher
Springer Science and Business Media LLC
Citation
Chen, W., Mazumder, R. & Samworth, R.J. A new computational framework for log-concave density estimation. Math. Prog. Comp. (2024).
Version: Final published version
ISSN
1867-2949
1867-2957