Rethinking Cross-Domain Sequential Recommendation under Open-World Assumptions
Author(s)
Xu, Wujiang; Wu, Qitian; Wang, Runzhong; Ha, Mingming; Ma, Qiongxu; Chen, Linxun; Han, Bing; Yan, Junchi; ... Show more Show less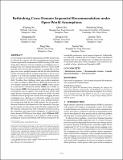
Download3589334.3645351.pdf (1.411Mb)
Publisher Policy
Publisher Policy
Article is made available in accordance with the publisher's policy and may be subject to US copyright law. Please refer to the publisher's site for terms of use.
Terms of use
Metadata
Show full item recordAbstract
Cross-Domain Sequential Recommendation (CDSR) methods aim to tackle the data sparsity and cold-start problems present in Single-Domain Sequential Recommendation (SDSR). Existing CDSR works design their elaborate structures relying on overlapping users to propagate the cross-domain information. However, current CDSR methods make closed-world assumptions, assuming fully overlapping users across multiple domains and that the data distribution remains unchanged from the training environment to the test environment. As a result, these methods typically result in lower performance on online real-world platforms due to the data distribution shifts. To address these challenges under open-world assumptions, we design an Adaptive Multi-Interest Debiasing framework for cross-domain sequential recommendation (AMID), which consists of a multi-interest information module (MIM) and a doubly robust estimator (DRE). Our framework is adaptive for open-world environments and can improve the model of most off-the-shelf single-domain sequential backbone models for CDSR. Our MIM establishes interest groups that consider both overlapping and non-overlapping users, allowing us to effectively explore user intent and explicit interest. To alleviate biases across multiple domains, we developed the DRE for the CDSR methods. We also provide a theoretical analysis that demonstrates the superiority of our proposed estimator in terms of bias and tail bound, compared to the IPS estimator used in previous work. To promote related research in the community under open-world assumptions, we collected an industry financial CDSR dataset from Alipay, called "MYbank-CDR". Extensive offline experiments on four industry CDSR scenarios including the Amazon and MYbank-CDR datasets demonstrate the remarkable performance of our proposed approach. Additionally, we conducted a standard A/B test on Alipay, a large-scale financial platform with over one billion users, to validate the effectiveness of our model under open-world assumptions. Code and dataset are available at https://github.com/WujiangXu/AMID.
Description
WWW '24: Proceedings of the ACM on Web Conference May 13–17, 2024, Singapore, Singapore
Date issued
2024-05-13Publisher
ACM
Citation
Xu, Wujiang, Wu, Qitian, Wang, Runzhong, Ha, Mingming, Ma, Qiongxu et al. 2024. "Rethinking Cross-Domain Sequential Recommendation under Open-World Assumptions."
Version: Final published version
ISBN
979-8-4007-0171-9