Smooth Anonymity for Sparse Graphs
Author(s)
Epasto, Alessandro; Esfandiari, Hossein; Mirrokni, Vahab; Munoz Medina, Andres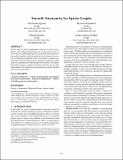
Download3589335.3651561.pdf (1.559Mb)
Publisher Policy
Publisher Policy
Article is made available in accordance with the publisher's policy and may be subject to US copyright law. Please refer to the publisher's site for terms of use.
Terms of use
Metadata
Show full item recordAbstract
In this work, we aim to manipulate and share an entire sparse dataset with a third party privately. As our first main result, we prove that any differentially private mechanism that maintains a reasonable similarity with the initial dataset is doomed to have a very weak privacy guarantee. Next, we consider a variation of k-anonymity, which we call smooth-k-anonymity, and design a simple large-scale algorithm that efficiently provides smooth-k-anonymity. We further perform an empirical evaluation and show that our algorithm improves the performance in downstream machine learning tasks on anonymized data.
Description
WWW '24: Companion Proceedings of the ACM on Web Conference May 13–17, 2024, Singapore, Singapore
Date issued
2024-05-13Department
Massachusetts Institute of Technology. Computer Science and Artificial Intelligence LaboratoryPublisher
ACM
Citation
Epasto, Alessandro, Esfandiari, Hossein, Mirrokni, Vahab and Munoz Medina, Andres. 2024. "Smooth Anonymity for Sparse Graphs."
Version: Final published version
ISBN
979-8-4007-0172-6