Robust Recovery for Stochastic Block Models, Simplified and Generalized
Author(s)
Mohanty, Sidhanth; Raghavendra, Prasad; Wu, David X.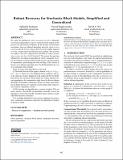
Download3618260.3649761.pdf (212.5Kb)
Publisher with Creative Commons License
Publisher with Creative Commons License
Creative Commons Attribution
Terms of use
Metadata
Show full item recordAbstract
We study the problem of robust community recovery: efficiently recovering communities in sparse stochastic block models in the presence of adversarial corruptions. In the absence of adversarial corruptions, there are efficient algorithms when the signal-to-noise ratio exceeds the Kesten–Stigum (KS) threshold, widely believed to be the computational threshold for this problem. The question we study is: does the computational threshold for robust community recovery also lie at the KS threshold? We answer this question affirmatively, providing an algorithm for robust community recovery for arbitrary stochastic block models on any constant number of communities, generalizing the work of Ding, d’Orsi, Nasser & Steurer on an efficient algorithm above the KS threshold in the case of 2-community block models. There are three main ingredients to our work: (1) The Bethe Hessian of the graph is defined as HG(t) ≜ (DG−I)t2 − AGt + I where DG is the diagonal matrix of degrees and AG is the adjacency matrix. Empirical work suggested that the Bethe Hessian for the stochastic block model has outlier eigenvectors corresponding to the communities right above the Kesten-Stigum threshold. We formally confirm the existence of outlier eigenvalues for the Bethe Hessian, by explicitly constructing outlier eigenvectors from the community vectors. (2) We develop an algorithm for a variant of robust PCA on sparse matrices. Specifically, an algorithm to partially recover top eigenspaces from adversarially corrupted sparse matrices under mild delocalization constraints. (3) A rounding algorithm to turn vector assignments of vertices into a community assignment, inspired by the algorithm of Charikar & Wirth for 2XOR.
Description
STOC ’24, June 24–28, 2024, Vancouver, BC, Canada
Date issued
2024-06-10Department
Massachusetts Institute of Technology. Computer Science and Artificial Intelligence LaboratoryPublisher
ACM
Citation
Mohanty, Sidhanth, Raghavendra, Prasad and Wu, David X. 2024. "Robust Recovery for Stochastic Block Models, Simplified and Generalized."
Version: Final published version
ISBN
979-8-4007-0383-6
Collections
The following license files are associated with this item: