On the geometry and refined rate of primal–dual hybrid gradient for linear programming
Author(s)
Lu, Haihao; Yang, Jinwen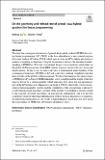
Download10107_2024_Article_2109.pdf (1.051Mb)
Publisher with Creative Commons License
Publisher with Creative Commons License
Creative Commons Attribution
Terms of use
Metadata
Show full item recordAbstract
We study the convergence behaviors of primal–dual hybrid gradient (PDHG) for solving linear programming (LP). PDHG is the base algorithm of a new general-purpose first-order method LP solver, PDLP, which aims to scale up LP by taking advantage of modern computing architectures. Despite its numerical success, the theoretical understanding of PDHG for LP is still very limited; the previous complexity result relies on the global Hoffman constant of the KKT system, which is known to be very loose and uninformative. In this work, we aim to develop a fundamental understanding of the convergence behaviors of PDHG for LP and to develop a refined complexity rate that does not rely on the global Hoffman constant. We show that there are two major stages of PDHG for LP: in Stage I, PDHG identifies active variables and the length of the first stage is driven by a certain quantity which measures how close the non-degeneracy part of the LP instance is to degeneracy; in Stage II, PDHG effectively solves a homogeneous linear inequality system, and the complexity of the second stage is driven by a well-behaved local sharpness constant of the system. This finding is closely related to the concept of partial smoothness in non-smooth optimization, and it is the first complexity result of finite time identification without the non-degeneracy assumption. An interesting implication of our results is that degeneracy itself does not slow down the convergence of PDHG for LP, but near-degeneracy does.
Date issued
2024-07-17Department
Sloan School of ManagementJournal
Mathematical Programming
Publisher
Springer Science and Business Media LLC
Citation
Lu, H., Yang, J. On the geometry and refined rate of primal–dual hybrid gradient for linear programming. Math. Program. (2024).
Version: Final published version
ISSN
0025-5610
1436-4646