A data-driven approach to mapping multidimensional poverty at residential block level in Mexico
Author(s)
Zea-Ortiz, Marivel; Vera, Pablo; Salas, Joaquín; Manduchi, Roberto; Villaseñor, Elio; Figueroa, Alejandra; Suárez, Ranyart R.; ... Show more Show less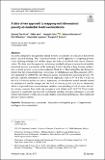
Download10668_2024_Article_5230.pdf (2.347Mb)
Publisher with Creative Commons License
Publisher with Creative Commons License
Creative Commons Attribution
Terms of use
Metadata
Show full item recordAbstract
Accurate, inexpensive and granular human poverty assessments are critical for data-driven policy decision-making. This research proposes a novel approach to computing poverty scores utilizing multispectral satellite images and indices calculated from census reference values. We show how this approach can leverage standard and sparse survey-based multidimensional poverty assessments at the municipal level to develop a deep learning architecture to obtain poverty scores at the residential block level. This method has the distinctive feature that the obtained inference corresponds to Multidimensional Measurement of Poverty generated by CONEVAL, the Mexican agency responsible for measuring poverty. We provide a reliable alternative to survey-based approaches with an 𝑅2
of 0.802±0.022
for the lack of housing quality and spaces dimension. A convolutional neural network trained on multispectral satellite images and the lack of housing quality and spaces dimension, which is regressed from census reference variables corresponding to lack of water, electricity, sewage, concrete floor, toilet and occupancy level obtains an 𝑅2
of 0.753. These results represent a significant step forward in including machine learning techniques to provide reliable information at reduced costs and a higher spatiotemporal frequency than traditional person-to-person surveys.
Date issued
2024-07-21Department
Massachusetts Institute of Technology. Department of Earth, Atmospheric, and Planetary SciencesJournal
Environment, Development and Sustainability
Publisher
Springer Science and Business Media LLC
Citation
Zea-Ortiz, M., Vera, P., Salas, J. et al. A data-driven approach to mapping multidimensional poverty at residential block level in Mexico. Environ Dev Sustain (2024).
Version: Final published version
ISSN
1573-2975