SGD-based optimization in modeling combustion kinetics: Case studies in tuning mechanistic and hybrid kinetic models
Author(s)
Ji, Weiqi; Su, Xingyu; Pang, Bin; Li, Yujuan; Ren, Zhuyin; Deng, Sili; ... Show more Show less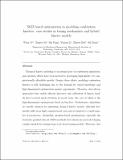
DownloadAccepted version (1.039Mb)
Open Access Policy
Open Access Policy
Creative Commons Attribution-Noncommercial-Share Alike
Terms of use
Metadata
Show full item recordAbstract
Chemical kinetic modeling is an integral part of combustion simulation, and extensive efforts have been devoted to developing high-fidelity yet computationally affordable models. Despite these efforts, modeling combustion kinetics is still challenging due to the demand for expert knowledge and high dimensional optimization against experiments. Therefore, data-driven approaches that enable efficient discovery and calibration of kinetic models have received much attention in recent years, the core of which is the high-dimensional optimization based on big data. Evolutionary algorithms are usually adopted for optimizing chemical kinetic models, although they usually suffer from high computational costs and are limited to a small number of parameters. Meanwhile, gradient-based optimizations, especially the stochastic gradient descent (SGD) methods, have shown success in developing complex models by training large-scale deep learning models. Therefore, this work explores the applications of SGD-based optimizations in tuning mechanistic kinetic models and learning hybrid kinetic models. We first showed that SGD-based optimizations could substantially save computational cost compared to evolutionary algorithms when the number of kinetic parameters in mechanistic models reached about one hundred. We then demonstrated that the SGD-based optimization enabled us to use a neural network model to represent the pyrolysis of the Hybrid Chemistry and optimize the associated hundreds of weights in the neural network. These proof-of-concept studies showed that the SGD-based optimization is more efficient than evolutionary algorithms, is a promising approach for developing chemical kinetic models with high dimensional parameters, and is capable of developing hybrid mechanistic-machine learning kinetic models.
Date issued
2022-09Department
Massachusetts Institute of Technology. Department of Mechanical EngineeringJournal
Fuel
Publisher
Elsevier BV
Citation
Ji, Weiqi, Su, Xingyu, Pang, Bin, Li, Yujuan, Ren, Zhuyin et al. 2022. "SGD-based optimization in modeling combustion kinetics: Case studies in tuning mechanistic and hybrid kinetic models." Fuel, 324.
Version: Author's final manuscript