Content Analysis of E-Participation Platforms in Taiwan with Topic Modeling: How to Train and Evaluate Neural Topic Models?
Author(s)
Sontheimer, Moritz; Fahlbusch, Jonas; Chou, Shuo-Yan; Kuo, Yu-Lin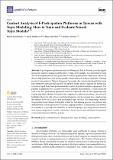
Downloadapplsci-15-02263.pdf (1.102Mb)
Publisher with Creative Commons License
Publisher with Creative Commons License
Creative Commons Attribution
Terms of use
Metadata
Show full item recordAbstract
E-participation platforms, such as iVoting and Join in Taiwan, provide digital spaces for citizens to engage in deliberation, voting, and oversight. As a forerunner in Asia, Taiwan has implemented these platforms to enhance participatory democracy. However, there is still limited research on the specific content debated on these platforms. Utilising recent advancements in Natural Language Processing, the content of proposals that users have submitted between 2015 and 2025 is explored. In this study, a pipeline for mining text corpora scraped from these platforms in the context of political analysis is proposed. The pipeline is applied to two datasets which have different characteristics. A topic model for each of the two platforms is generated and later evaluated with OCTIS (Optimizing and Comparing Topic Models Is Simple) and compared to different baselines. Our research highlights the trade-offs between model performance and processing time, emphasizing the balance between accuracy and meaningful topic creation. By integrating a translation pipeline from Chinese to English within the text-mining process, our method also demonstrates a solid approach to overcome language barriers. Consequently, our method is adaptable to e-participation platforms in various languages, providing decision-makers with a more comprehensive tool to understand citizens’ needs and enabling the formulation of more informed and effective policies.
Date issued
2025-02-20Journal
Applied Sciences
Publisher
Multidisciplinary Digital Publishing Institute
Citation
Sontheimer, M.; Fahlbusch, J.; Chou, S.-Y.; Kuo, Y.-L. Content Analysis of E-Participation Platforms in Taiwan with Topic Modeling: How to Train and Evaluate Neural Topic Models? Appl. Sci. 2025, 15, 2263.
Version: Final published version