The P-Norm Push: A Simple Convex Ranking Algorithm that Concentrates at the Top of the List
Author(s)
Rudin, Cynthia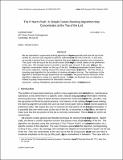
DownloadRudin_The P-Norm Push.PDF (1.094Mb)
PUBLISHER_POLICY
Publisher Policy
Article is made available in accordance with the publisher's policy and may be subject to US copyright law. Please refer to the publisher's site for terms of use.
Terms of use
Metadata
Show full item recordAbstract
We are interested in supervised ranking algorithms that perform especially well near the top of the
ranked list, and are only required to perform sufficiently well on the rest of the list. In this work,
we provide a general form of convex objective that gives high-scoring examples more importance.
This “push” near the top of the list can be chosen arbitrarily large or small, based on the preference
of the user. We choose ℓp-norms to provide a specific type of push; if the user sets p larger, the
objective concentrates harder on the top of the list. We derive a generalization bound based on
the p-norm objective, working around the natural asymmetry of the problem. We then derive a
boosting-style algorithm for the problem of ranking with a push at the top. The usefulness of the
algorithm is illustrated through experiments on repository data. We prove that the minimizer of the
algorithm’s objective is unique in a specific sense. Furthermore, we illustrate how our objective is
related to quality measurements for information retrieval.
Date issued
2009-10Department
Sloan School of ManagementJournal
Journal of Machine Learning Research
Publisher
MIT Press
Citation
Rudin, Cynthia."The P-Norm Push: A Simple Convex Ranking Algorithm that Concentrates at the Top of the List." Journal of Machine Learning Research 10 (2009) 2233-2271. ©2009 Cynthia Rudin.
Version: Final published version
ISSN
1533-7928)
1532-4435
Keywords
information retrieval, ROC, generalization bounds, RankBoost, ranking