Beyond local optimality: An improved approach to hybrid model learning
Author(s)
Gil, Stephanie; Williams, Brian Charles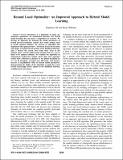
DownloadGil-2009-Beyond local optimality an improved approach to hybrid model learning.pdf (2.723Mb)
PUBLISHER_POLICY
Publisher Policy
Article is made available in accordance with the publisher's policy and may be subject to US copyright law. Please refer to the publisher's site for terms of use.
Terms of use
Metadata
Show full item recordAbstract
Local convergence is a limitation of many optimization approaches for multimodal functions. For hybrid model learning, this can mean a compromise in accuracy. We develop an approach for learning the model parameters of hybrid discrete-continuous systems that avoids getting stuck in locally optimal solutions. We present an algorithm that implements this approach that 1) iteratively learns the locations and shapes of explored local maxima of the likelihood function, and 2) focuses the search away from these areas of the solution space, toward undiscovered maxima that are a priori likely to be optimal solutions. We evaluate the algorithm on autonomous underwater vehicle (AUV) data. Our aggregate results show reduction in distance to the global maximum by 16% in 10 iterations, averaged over 100 trials, and iterative increase in log-likelihood value of learned model parameters, demonstrating the ability of the algorithm to guide the search toward increasingly better optima of the likelihood function, avoiding local convergence.
Date issued
2010-01Department
Massachusetts Institute of Technology. Department of Aeronautics and AstronauticsJournal
Proceedings of the 48th IEEE Conference on Decision and Control, 2009 held jointly with the 2009 28th Chinese Control Conference. CDC/CCC 2009
Publisher
Institute of Electrical and Electronics Engineers
Citation
Gil, S., and B. Williams. “Beyond local optimality: An improved approach to hybrid model learning.” Decision and Control, 2009 held jointly with the 2009 28th Chinese Control Conference. CDC/CCC 2009. Proceedings of the 48th IEEE Conference on. 2009. 3938-3945. © 2010 Institute of Electrical and Electronics Engineers.
Version: Final published version
Other identifiers
INSPEC Accession Number: 11149477
ISBN
978-1-4244-3871-6
ISSN
0191-2216