Exploring functional connectivity in fMRI via clustering
Author(s)
Venkataraman, Archana; Van Dijk, Koene R. A.; Buckner, Randy L.; Golland, Polina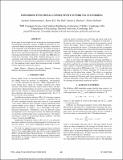
DownloadGolland_Exploring functional.pdf (187.5Kb)
PUBLISHER_POLICY
Publisher Policy
Article is made available in accordance with the publisher's policy and may be subject to US copyright law. Please refer to the publisher's site for terms of use.
Terms of use
Metadata
Show full item recordAbstract
In this paper we investigate the use of data driven clustering methods for functional connectivity analysis in fMRI. In particular, we consider the k-means and spectral clustering algorithms as alternatives to the commonly used seed-based analysis. To enable clustering of the entire brain volume, we use the Nystrom Method to approximate the necessary spectral decompositions. We apply k-means, spectral clustering and seed-based analysis to resting-state fMRI data collected from 45 healthy young adults. Without placing any a priori constraints, both clustering methods yield partitions that are associated with brain systems previously identified via seed-based analysis. Our empirical results suggest that clustering provides a valuable tool for functional connectivity analysis.
Date issued
2009-04Department
Massachusetts Institute of Technology. Computer Science and Artificial Intelligence Laboratory; Massachusetts Institute of Technology. Department of Electrical Engineering and Computer ScienceJournal
IEEE International Conference on Acoustics, Speech and Signal Processing (ICASSP)
Publisher
Institute of Electrical and Electronics Engineers
Citation
Venkataraman, A. et al. “Exploring Functional Connectivity in fMRI via Clustering.” Acoustics, Speech and Signal Processing, 2009. ICASSP 2009. IEEE International Conference On. 2009. 441-444. Copyright © 2009, IEEE
Version: Final published version
Other identifiers
INSPEC Accession Number: 10700583
ISBN
978-1-4244-2353-8
ISSN
1520-6149