A data-driven approach for even prediction
Author(s)
Yuen, Jenny; Torralba, Antonio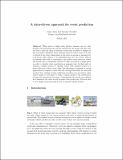
DownloadTorralba_A data.pdf (10.78Mb)
OPEN_ACCESS_POLICY
Open Access Policy
Creative Commons Attribution-Noncommercial-Share Alike
Terms of use
Metadata
Show full item recordAbstract
When given a single static picture, humans can not only
interpret the instantaneous content captured by the image, but also they
are able to infer the chain of dynamic events that are likely to happen in
the near future. Similarly, when a human observes a short video, it is easy
to decide if the event taking place in the video is normal or unexpected,
even if the video depicts a an unfamiliar place for the viewer. This is
in contrast with work in surveillance and outlier event detection, where
the models rely on thousands of hours of video recorded at a single place
in order to identify what constitutes an unusual event. In this work we
present a simple method to identify videos with unusual events in a
large collection of short video clips. The algorithm is inspired by recent
approaches in computer vision that rely on large databases. In this work
we show how, relying on large collections of videos, we can retrieve other
videos similar to the query to build a simple model of the distribution
of expected motions for the query. Consequently, the model can evaluate
how unusual is the video as well as make event predictions. We show how
a very simple retrieval model is able to provide reliable results.
Date issued
2010-09Department
Massachusetts Institute of Technology. Computer Science and Artificial Intelligence Laboratory; Massachusetts Institute of Technology. Department of Electrical Engineering and Computer ScienceJournal
ECCV'10 Proceedings of the 11th European Conference on Computer Vision: Part II
Publisher
Springer-Verlag
Citation
Yuen, Jenny, and Antonio Torralba. “A data-driven approach for event prediction.” Proceedings of the 11th European Conference on Computer Vision: Part II. Heraklion, Crete, Greece: Springer-Verlag, 2010. 707-720.
Version: Author's final manuscript
ISBN
978-3-642-15551-2