Consumer Credit-Risk Models Via Machine-Learning Algorithms
Author(s)
Khandani, Amir Ehsan; Kim, Adlar J.; Lo, Andrew W.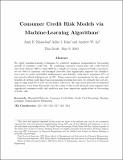
DownloadConsumer Credit Risk.pdf (6.178Mb)
OPEN_ACCESS_POLICY
Open Access Policy
Creative Commons Attribution-Noncommercial-Share Alike
Terms of use
Metadata
Show full item recordAbstract
We apply machine-learning techniques to construct nonlinear nonparametric forecasting models of consumer credit risk. By combining customer transactions and credit bureau data from January 2005 to April 2009 for a sample of a major commercial bank’s customers, we are able to construct out-of-sample forecasts that significantly improve the classification rates of credit-card-holder delinquencies and defaults, with linear regression R2’s of forecasted/realized delinquencies of 85%. Using conservative assumptions for the costs and benefits of cutting credit lines based on machine-learning forecasts, we estimate the cost savings to range from 6% to 25% of total losses. Moreover, the time-series patterns of estimated delinquency rates from this model over the course of the recent financial crisis suggest that aggregated consumer credit-risk analytics may have important applications in forecasting systemic risk.
Date issued
2010-06Department
Massachusetts Institute of Technology. Computer Science and Artificial Intelligence Laboratory; Sloan School of Management; Sloan School of Management. Laboratory for Financial EngineeringJournal
Journal of Banking and Finance
Publisher
Elsevier B.V.
Citation
Khandani, Amir E., Adlar J. Kim, and Andrew W. Lo. “Consumer credit-risk models via machine-learning algorithms☆.” Journal of Banking & Finance 34 (2010): 2767-2787.
Version: Author's final manuscript
ISSN
0378-4266