Neural network Jacobian analysis for high-resolution profiling of the atmosphere
Author(s)
Blackwell, William J.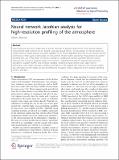
Download1687-6180-2012-71.pdf (485.8Kb)
OPEN_ACCESS_POLICY
Open Access Policy
Creative Commons Attribution-Noncommercial-Share Alike
Terms of use
Metadata
Show full item recordAbstract
Neural networks have been widely used to provide retrievals of geophysical parameters from spectral radiance measurements made remotely by air-, ground-, and space-based sensors. The advantages of retrievals based on neural networks include speed of execution, simplicity of the trained algorithm, and ease of error analysis, and the proliferation of high quality training data sets derived from models and/or operational measurements has further facilitated their use. In this article, we provide examples of geophysical retrieval algorithms based on neural networks with a focus on Jacobian analysis. We examine a hypothetical 80-channel hyperspectral microwave atmospheric sounder (HyMAS) and construct examples comparing neural network water vapor retrieval performance with simple regressions. Jacobians (derivatives of the outputs with respect to the network weights and with respect to the inputs) are also presented and discussed. Finally, a discussion of the Jacobian operating points is provided.
Date issued
2012-03Department
Lincoln LaboratoryJournal
EURASIP Journal on Advances in Signal Processing
Publisher
Springer
Citation
Blackwell, William J. “Neural Network Jacobian Analysis for High-resolution Profiling of the Atmosphere.” EURASIP Journal on Advances in Signal Processing 2012.1 (2012): 71.
Version: Author's final manuscript
ISSN
1687-6172
1687-6180