Part and appearance sharing: Recursive compositional models for multi-view multi-object detection
Author(s)
Zhu, Long; Chen, Yuanhao; Torralba, Antonio; Freeman, William T.; Yuille, Alan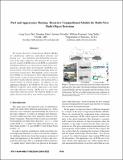
DownloadFreeman-Part and Appearance.pdf (1.605Mb)
PUBLISHER_POLICY
Publisher Policy
Article is made available in accordance with the publisher's policy and may be subject to US copyright law. Please refer to the publisher's site for terms of use.
Terms of use
Metadata
Show full item recordAbstract
We propose Recursive Compositional Models (RCMs) for simultaneous multi-view multi-object detection and parsing (e.g. view estimation and determining the positions of the object subparts). We represent the set of objects by a family of RCMs where each RCM is a probability distribution defined over a hierarchical graph which corresponds to a specific object and viewpoint. An RCM is constructed from a hierarchy of subparts/subgraphs which are learnt from training data. Part-sharing is used so that different RCMs are encouraged to share subparts/subgraphs which yields a compact representation for the set of objects and which enables efficient inference and learning from a limited number of training samples. In addition, we use appearance-sharing so that RCMs for the same object, but different viewpoints, share similar appearance cues which also helps efficient learning. RCMs lead to a multi-view multi-object detection system. We illustrate RCMs on four public datasets and achieve state-of-the-art performance.
Date issued
2010-08Department
Massachusetts Institute of Technology. Computer Science and Artificial Intelligence Laboratory; Massachusetts Institute of Technology. Department of Electrical Engineering and Computer ScienceJournal
2010 IEEE Conference on Computer Vision and Pattern Recognition
Publisher
Institute of Electrical and Electronics Engineers (IEEE)
Citation
Detection, Multi- et al. “Part and Appearance Sharing: Recursive Compositional Models for Multi-view.” IEEE, 2010. 1919–1926. © Copyright 2010 IEEE
Version: Final published version
ISBN
978-1-4244-6984-0
ISSN
1063-6919