Fast Averaging
Author(s)
Bodas, Shreeshankar; Shah, Devavrat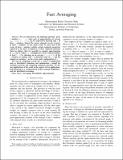
DownloadShah_Fast averaging.pdf (151.5Kb)
OPEN_ACCESS_POLICY
Open Access Policy
Creative Commons Attribution-Noncommercial-Share Alike
Terms of use
Metadata
Show full item recordAbstract
We are interested in the following question: given n numbers x[subscript 1], ..., x[subscript n], what sorts of approximation of average x[subscript ave] = 1overn (x[subscript 1] + ... + x[subscript n]) can be achieved by knowing only r of these n numbers. Indeed the answer depends on the variation in these n numbers. As the main result, we show that if the vector of these n numbers satisfies certain regularity properties captured in the form of finiteness of their empirical moments (third or higher), then it is possible to compute approximation of x[subscript ave] that is within 1 ±ε multiplicative factor with probability at least 1 - δ by choosing, on an average, r = r(ε, δ, σ) of the n numbers at random with r is dependent only on ε, δ and the amount of variation σ in the vector and is independent of n. The task of computing average has a variety of applications such as distributed estimation and optimization, a model for reaching consensus and computing symmetric functions. We discuss implications of the result in the context of two applications: load-balancing in a computational facility running MapReduce, and fast distributed averaging.
Date issued
2011-10Department
Massachusetts Institute of Technology. Department of Electrical Engineering and Computer Science; Massachusetts Institute of Technology. Laboratory for Information and Decision SystemsJournal
IEEE International Symposium on Information Theory Proceedings 2011 (ISIT)
Publisher
Institute of Electrical and Electronics Engineers (IEEE)
Citation
Bodas, Shreeshankar, and Devavrat Shah. “Fast Averaging.” IEEE International Symposium on Information Theory Proceedings 2011 (ISIT). 2153–2157.
Version: Author's final manuscript
ISBN
978-1-4577-0594-6
978-1-4577-0596-0
ISSN
2157-8095