The convex algebraic geometry of linear inverse problems
Author(s)
Chandrasekaran, Venkat; Recht, Benjamin; Parrilo, Pablo A.; Willsky, Alan S.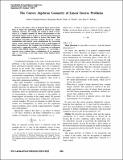
DownloadParrilo_The convex algebraic.pdf (316.6Kb)
PUBLISHER_POLICY
Publisher Policy
Article is made available in accordance with the publisher's policy and may be subject to US copyright law. Please refer to the publisher's site for terms of use.
Terms of use
Metadata
Show full item recordAbstract
We study a class of ill-posed linear inverse problems in which the underlying model of interest has simple algebraic structure. We consider the setting in which we have access to a limited number of linear measurements of the underlying model, and we propose a general framework based on convex optimization in order to recover this model. This formulation generalizes previous methods based on ℓ[subscript 1]-norm minimization and nuclear norm minimization for recovering sparse vectors and low-rank matrices from a small number of linear measurements. For example some problems to which our framework is applicable include (1) recovering an orthogonal matrix from limited linear measurements, (2) recovering a measure given random linear combinations of its moments, and (3) recovering a low-rank tensor from limited linear observations.
Date issued
2010-09Department
Massachusetts Institute of Technology. Department of Electrical Engineering and Computer Science; Massachusetts Institute of Technology. Laboratory for Information and Decision SystemsJournal
Proceedings of the 48th Annual Allerton Conference on Communication, Control, and Computing (Allerton), 2010
Publisher
Institute of Electrical and Electronics Engineers (IEEE)
Citation
Chandrasekaran, Venkat et al. “The Convex Algebraic Geometry of Linear Inverse Problems.” 48th Annual Allerton Conference on Communication, Control, and Computing (Allerton), 2010. 699–703. © Copyright 2010 IEEE
Version: Final published version
ISBN
978-1-4244-8215-3