Incremental proximal methods for large scale convex optimization
Author(s)
Bertsekas, Dimitri P.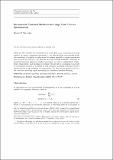
DownloadBertsekas-Incremental proximal.pdf (244.5Kb)
OPEN_ACCESS_POLICY
Open Access Policy
Creative Commons Attribution-Noncommercial-Share Alike
Terms of use
Metadata
Show full item recordAbstract
We consider the minimization of a sum∑m [over]i=1 fi (x) consisting of a large
number of convex component functions fi . For this problem, incremental methods
consisting of gradient or subgradient iterations applied to single components have
proved very effective. We propose new incremental methods, consisting of proximal
iterations applied to single components, as well as combinations of gradient, subgradient,
and proximal iterations. We provide a convergence and rate of convergence
analysis of a variety of such methods, including some that involve randomization in
the selection of components.We also discuss applications in a few contexts, including
signal processing and inference/machine learning.
Description
Laboratory for Information and Decision Systems Report LIDS-P-2847
Date issued
2011-06Department
Massachusetts Institute of Technology. Department of Electrical Engineering and Computer ScienceJournal
Mathematical Programming
Publisher
Springer-Verlag
Citation
Bertsekas, Dimitri P. “Incremental proximal methods for large scale convex optimization.” Mathematical Programming 129.2 (2011): 163-195.
Version: Author's final manuscript
ISSN
0025-5610
1436-4646