Inferring rankings using constrained sensing
Author(s)
Jagabathula, Srikanth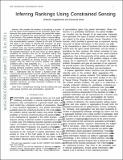
DownloadShah_Inferring rankings.pdf (336.2Kb)
OPEN_ACCESS_POLICY
Open Access Policy
Creative Commons Attribution-Noncommercial-Share Alike
Terms of use
Metadata
Show full item recordAbstract
We consider the problem of recovering a function over the space of permutations (or, the symmetric group) over n elements from given partial information; the partial information we consider is related to the group theoretic Fourier Transform of the function. This problem naturally arises in several settings such as ranked elections, multi-object tracking, ranking systems, and recommendation systems. Inspired by the work of Donoho and Stark in the context of discrete-time functions, we focus on non-negative functions with a sparse support (support size <;<; domain size). Our recovery method is based on finding the sparsest solution (through l[subscript 0] optimization) that is consistent with the available information. As the main result, we derive sufficient conditions for functions that can be recovered exactly from partial information through l[subscript 0] optimization. Under a natural random model for the generation of functions, we quantify the recoverability conditions by deriving bounds on the sparsity (support size) for which the function satisfies the sufficient conditions with a high probability as n → ∞. ℓ0 optimization is computationally hard. Therefore, the popular compressive sensing literature considers solving the convex relaxation, ℓ[subscript 1] optimization, to find the sparsest solution. However, we show that ℓ[subscript 1] optimization fails to recover a function (even with constant sparsity) generated using the random model with a high probability as n → ∞. In order to overcome this problem, we propose a novel iterative algorithm for the recovery of functions that satisfy the sufficient conditions. Finally, using an Information Theoretic framework, we study necessary conditions for exact recovery to be possible.
Date issued
2011-11Department
Massachusetts Institute of Technology. Department of Electrical Engineering and Computer ScienceJournal
IEEE Transactions on Information Theory
Publisher
Institute of Electrical and Electronics Engineers (IEEE)
Citation
Jagabathula, S.; Shah, D.; , "Inferring Rankings Using Constrained Sensing," Information Theory, IEEE Transactions on , vol.57, no.11, pp.7288-7306, Nov. 2011
Version: Author's final manuscript
ISSN
0018-9448