Consistent and efficient reconstruction of latent tree models
Author(s)
Choi, Myung Jin; Tan, Vincent Yan Fu; Anandkumar, Animashree; Willsky, Alan S.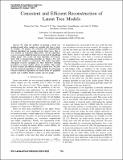
DownloadWillsky_Consistent and efficient.pdf (386.3Kb)
PUBLISHER_POLICY
Publisher Policy
Article is made available in accordance with the publisher's policy and may be subject to US copyright law. Please refer to the publisher's site for terms of use.
Terms of use
Metadata
Show full item recordAbstract
We study the problem of learning a latent tree graphical model where samples are available only from a subset of variables. We propose two consistent and computationally efficient algorithms for learning minimal latent trees, that is, trees without any redundant hidden nodes. Our first algorithm, recursive grouping, builds the latent tree recursively by identifying sibling groups. Our second and main algorithm, CLGrouping, starts with a pre-processing procedure in which a tree over the observed variables is constructed. This global step guides subsequent recursive grouping (or other latent-tree learning procedures) on much smaller subsets of variables. This results in more accurate and efficient learning of latent trees. We compare the proposed algorithms to other methods by performing extensive numerical experiments on various latent tree graphical models such as hidden Markov models and star graphs.
Date issued
2010-09Department
Massachusetts Institute of Technology. Department of Electrical Engineering and Computer Science; Massachusetts Institute of Technology. Laboratory for Information and Decision SystemsJournal
Proceedings of the 48th Annual Allerton Conference on Communication, Control, and Computing (Allerton), 2010
Publisher
Institute of Electrical and Electronics Engineers (IEEE)
Citation
Choi, Myung Jin et al. “Consistent and Efficient Reconstruction of Latent Tree Models.” 48th Annual Allerton Conference on Communication, Control, and Computing (Allerton), 2010. 719–725. ©2010 IEEE
Version: Final published version
ISBN
978-1-4244-8215-3