Nonparametric Bayesian identification of jump systems with sparse dependencies
Author(s)
Fox, Emily Beth; Sudderth, Erik B.; Jordan, Michael I.; Willsky, Alan S.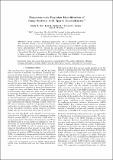
DownloadWillsky_Nonparametric Bayesian.pdf (609.1Kb)
OPEN_ACCESS_POLICY
Open Access Policy
Creative Commons Attribution-Noncommercial-Share Alike
Terms of use
Metadata
Show full item recordAbstract
Many nonlinear dynamical phenomena can be effectively modeled by a system that switches among a set of conditionally linear dynamical modes. We consider two such Markov jump linear systems: the switching linear dynamical system (SLDS) and the switching vector autoregressive (S-VAR) process. In this paper, we present a nonparametric Bayesian approach to identifying an unknown number of persistent, smooth dynamical modes by utilizing a hierarchical Dirichlet process prior. We additionally employ automatic relevance determination to infer a sparse set of dynamic dependencies. The utility and flexibility of our models are demonstrated on synthetic data and a set of honey bee dances.
Date issued
2009-07Department
Massachusetts Institute of Technology. Department of Electrical Engineering and Computer ScienceJournal
15th Symposium on System Identification, SYSID 2009
Publisher
International Federation of Automatic Control (IFAC)
Citation
Emily, Fox. “Nonparametric Bayesian Identification of Jump Systems with Sparse Dependencies.” Ed. Walter Eric. 15th Symposium on System Identification, SYSID 2009. 1591–1596. © 2009 IFAC
Version: Author's final manuscript
ISBN
9783902661470
ISSN
14746670