Least Squares After Model Selection in High-dimensional Sparse Models
Author(s)
Belloni, Alexandre; Chernozhukov, Victor V.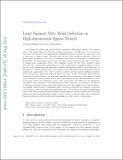
DownloadChernozhukov_Least squares.pdf (486.7Kb)
OPEN_ACCESS_POLICY
Open Access Policy
Creative Commons Attribution-Noncommercial-Share Alike
Terms of use
Metadata
Show full item recordAbstract
We study post-model selection estimators which apply ordinary least squares (ols) to the model selected by first-step penalized estimators. It is well known that lasso can estimate the nonparametric regression function at nearly the oracle rate, and is thus hard to improve upon. We show that ols post lasso estimator performs at least as well as lasso in terms of the rate of convergence, and has the advantage of a smaller bias. Remarkably, this performance occurs even if the lasso-based model selection "fails" in the sense of missing some components of the "true" regression model. By the "true" model we mean here the best $s$-dimensional approximation to the nonparametric regression function chosen by the oracle. Furthermore, ols post lasso estimator can perform strictly better than lasso, i.e. a strictly faster rate of convergence, if the lasso-based model selection correctly includes all components of the "true" model as a subset and also achieves sufficient sparsity. In the extreme case, when lasso perfectly selects the "true" model, the ols post lasso estimator becomes the oracle estimator. An important ingredient in our analysis is a new sparsity bound on the dimension of the model selected by lasso which guarantees that this dimension is at most of the same order as the dimension of the "true" model. Moreover, our analysis is not limited to the lasso estimator acting as selector in the first step, but also applies to any other estimator, for example various forms of thresholded lasso, with good rates and good sparsity properties. Our analysis covers both traditional thresholding and a new practical, data-driven thresholding scheme that induces maximal sparsity subject to maintaining a certain goodness-of-fit. The latter scheme has theoretical guarantees similar to those of lasso or ols post lasso, but it dominates these procedures in a wide variety of experiments.
Description
http://arxiv.org/abs/1001.0188
Date issued
2013-05Department
Massachusetts Institute of Technology. Department of EconomicsJournal
Bernoulli
Publisher
Bernoulli Society for Mathematical Statistics and Probability
Citation
Belloni, Alexandre and Victor V. Chernozhukov. "Least Squares After Model Selection in High-dimensional Sparse Models." Bernoulli, Vol. 19, No. 2, May 2013.
Version: Author's final manuscript
ISSN
1350-7265