A Brain-Machine Interface for Control of Medically-Induced Coma
Author(s)
Shanechi, Maryam M.; Chemali, Jessica J.; Liberman, Max; Solt, Ken; Brown, Emery N.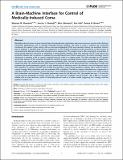
DownloadBrown_A Brain-Machine.pdf (1.170Mb)
PUBLISHER_CC
Publisher with Creative Commons License
Creative Commons Attribution
Terms of use
Metadata
Show full item recordAbstract
Medically-induced coma is a drug-induced state of profound brain inactivation and unconsciousness used to treat refractory intracranial hypertension and to manage treatment-resistant epilepsy. The state of coma is achieved by continually monitoring the patient's brain activity with an electroencephalogram (EEG) and manually titrating the anesthetic infusion rate to maintain a specified level of burst suppression, an EEG marker of profound brain inactivation in which bursts of electrical activity alternate with periods of quiescence or suppression. The medical coma is often required for several days. A more rational approach would be to implement a brain-machine interface (BMI) that monitors the EEG and adjusts the anesthetic infusion rate in real time to maintain the specified target level of burst suppression. We used a stochastic control framework to develop a BMI to control medically-induced coma in a rodent model. The BMI controlled an EEG-guided closed-loop infusion of the anesthetic propofol to maintain precisely specified dynamic target levels of burst suppression. We used as the control signal the burst suppression probability (BSP), the brain's instantaneous probability of being in the suppressed state. We characterized the EEG response to propofol using a two-dimensional linear compartment model and estimated the model parameters specific to each animal prior to initiating control. We derived a recursive Bayesian binary filter algorithm to compute the BSP from the EEG and controllers using a linear-quadratic-regulator and a model-predictive control strategy. Both controllers used the estimated BSP as feedback. The BMI accurately controlled burst suppression in individual rodents across dynamic target trajectories, and enabled prompt transitions between target levels while avoiding both undershoot and overshoot. The median performance error for the BMI was 3.6%, the median bias was -1.4% and the overall posterior probability of reliable control was 1 (95% Bayesian credibility interval of [0.87, 1.0]). A BMI can maintain reliable and accurate real-time control of medically-induced coma in a rodent model suggesting this strategy could be applied in patient care.
Date issued
2013-10Department
Institute for Medical Engineering and Science; Massachusetts Institute of Technology. Department of Brain and Cognitive SciencesJournal
PLoS Computational Biology
Publisher
Public Library of Science
Citation
Shanechi, Maryam M., Jessica J. Chemali, Max Liberman, Ken Solt, and Emery N. Brown. “A Brain-Machine Interface for Control of Medically-Induced Coma.” Edited by Olaf Sporns. PLoS Computational Biology 9, no. 10 (October 31, 2013): e1003284.
Version: Final published version
ISSN
1553-7358
1553-734X