SiblingRivalry: Online Autotuning Through Local Competitions
Author(s)
Ansel, Jason Andrew; Pacula, Maciej; Wong, Yee Lok; Chan, Cy; Olszewski, Marek Krystyn; O'Reilly, Una-May; Amarasinghe, Saman P.; ... Show more Show less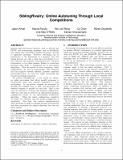
DownloadSibling rivalry.pdf (375.2Kb)
OPEN_ACCESS_POLICY
Open Access Policy
Creative Commons Attribution-Noncommercial-Share Alike
Terms of use
Metadata
Show full item recordAbstract
Modern high performance libraries, such as ATLAS and FFTW, and programming languages, such as PetaBricks, have shown that autotuning computer programs can lead to significant speedups. However, autotuning can be burdensome to the deployment of a program, since the tuning process can take a long time and should be re-run whenever the program, microarchitecture, execution environment, or tool chain changes. Failure to re-autotune programs often leads to widespread use of sub-optimal algorithms. With the growth of cloud computing, where computations can run in environments with unknown load and migrate between different (possibly unknown) microarchitectures, the need for online autotuning has become increasingly important.
We present SiblingRivalry, a new model for always-on online autotuning that allows parallel programs to continuously adapt and optimize themselves to their environment. In our system, requests are processed by dividing the available cores in half, and processing two identical requests in parallel on each half. Half of the cores are devoted to a known safe program configuration, while the other half are used for an experimental program configuration chosen by our self-adapting evolutionary algorithm. When the faster configuration completes, its results are returned, and the slower configuration is terminated. Over time, this constant experimentation allows programs to adapt to changing dynamic environments and often outperform the original algorithm that uses the entire system.
Date issued
2012-10Department
Massachusetts Institute of Technology. Computer Science and Artificial Intelligence LaboratoryJournal
Proceedings of the 2012 international conference on Compilers, architectures and synthesis for embedded systems - CASES '12
Publisher
Association for Computing Machinery
Citation
Ansel, Jason, Maciej Pacula, Yee Lok Wong, Cy Chan, Marek Olszewski, Una-May O’Reilly, and Saman Amarasinghe. “Siblingrivalry: Online Autotuning Through Local Competitions.” In Proceedings of the 2012 International Conference on Compilers, Architectures and Synthesis for Embedded Systems - CASES ’12 (2012), October 7-12, 2012, Tampere, Finland.
Version: Author's final manuscript
ISBN
9781450314244
1450314244