Counter-Driven Regression for Label Inference in Atlas-Based Segmentation
Author(s)
Wachinger, Christian; Sharp, Gregory C.; Golland, Polina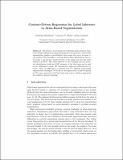
DownloadPaper366.pdf (809.9Kb)
OPEN_ACCESS_POLICY
Open Access Policy
Creative Commons Attribution-Noncommercial-Share Alike
Metadata
Show full item recordAbstract
We present a novel method for inferring tissue labels in atlas-based image segmentation using Gaussian process regression. Atlas-based segmentation results in probabilistic label maps that serve as input to our method. We introduce a contour-driven prior distribution over label maps to incorporate image features of the input scan into the label inference problem. The mean function of the Gaussian process posterior distribution yields the MAP estimate of the label map and is used in the subsequent voting. We demonstrate improved segmentation accuracy when our approach is combined with two different patch-based segmentation techniques. We focus on the segmentation of parotid glands in CT scans of patients with head and neck cancer, which is important for radiation therapy planning.
Date issued
2013-09Department
Massachusetts Institute of Technology. Computer Science and Artificial Intelligence Laboratory; Massachusetts Institute of Technology. Department of Electrical Engineering and Computer ScienceJournal
Medical Image Computing and Computer-Assisted Intervention – MICCAI 2013
Publisher
Springer-Verlag
Citation
Wachinger, Christian, Gregory C. Sharp, and Polina Golland. “Contour-Driven Regression for Label Inference in Atlas-Based Segmentation.” Medical Image Computing and Computer-Assisted Intervention – MICCAI 2013. Ed. Kensaku Mori et al. Vol. 8151. Springer Berlin Heidelberg, 2013. 211–218. Lecture Notes in Computer Science.
Version: Author's final manuscript
ISBN
978-3-642-40759-8
ISSN
978-3-642-40760-4
0302-9743