Spectral Label Fusion
Author(s)
Wachinger, Christian; Golland, Polina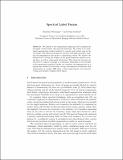
Downloadpaper264.pdf (714.5Kb)
OPEN_ACCESS_POLICY
Open Access Policy
Creative Commons Attribution-Noncommercial-Share Alike
Terms of use
Metadata
Show full item recordAbstract
We present a new segmentation approach that combines the strengths of label fusion and spectral clustering. The result is an atlas-based segmentation method guided by contour and texture cues in the test image. This offers advantages for datasets with high variability, making the segmentation less prone to registration errors. We achieve the integration by letting the weights of the graph Laplacian depend on image data, as well as atlas-based label priors. The extracted contours are converted to regions, arranged in a hierarchy depending on the strength of the separating boundary. Finally, we construct the segmentation by a region-wise, instead of voxel-wise, voting, increasing the robustness. Our experiments on cardiac MRI show a clear improvement over majority voting and intensity-weighted label fusion.
Date issued
2012-10Department
Massachusetts Institute of Technology. Computer Science and Artificial Intelligence LaboratoryJournal
Medical Image Computing and Computer-Assisted Intervention – MICCAI 2012
Publisher
Springer-Verlag
Citation
Wachinger, Christian, and Polina Golland. “Spectral Label Fusion.” Lecture Notes in Computer Science (2012): 410–417.
Version: Author's final manuscript
ISBN
978-3-642-33453-5
978-3-642-33454-2
ISSN
0302-9743
1611-3349