Adaptive Construction of Surrogates for the Bayesian Solution of Inverse Problems
Author(s)
Li, Jinglai; Marzouk, Youssef M.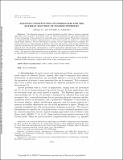
DownloadLi-2014-Adaptive constructio.pdf (823.0Kb)
PUBLISHER_POLICY
Publisher Policy
Article is made available in accordance with the publisher's policy and may be subject to US copyright law. Please refer to the publisher's site for terms of use.
Terms of use
Metadata
Show full item recordAbstract
The Bayesian approach to inverse problems typically relies on posterior sampling approaches, such as Markov chain Monte Carlo, for which the generation of each sample requires one or more evaluations of the parameter-to-observable map or forward model. When these evaluations are computationally intensive, approximations of the forward model are essential to accelerating sample-based inference. Yet the construction of globally accurate approximations for nonlinear forward models can be computationally prohibitive and in fact unnecessary, as the posterior distribution typically concentrates on a small fraction of the support of the prior distribution. We present a new approach that uses stochastic optimization to construct polynomial approximations over a sequence of distributions adaptively determined from the data, eventually concentrating on the posterior distribution. The approach yields substantial gains in efficiency and accuracy over prior-based surrogates, as demonstrated via application to inverse problems in partial differential equations.
Date issued
2014-06Department
Massachusetts Institute of Technology. Department of Aeronautics and AstronauticsJournal
SIAM Journal on Scientific Computing
Publisher
Society for Industrial and Applied Mathematics
Citation
Li, Jinglai, and Youssef M. Marzouk. “Adaptive Construction of Surrogates for the Bayesian Solution of Inverse Problems.” SIAM Journal on Scientific Computing 36, no. 3 (January 2014): A1163–A1186. © 2014, Society for Industrial and Applied Mathematics
Version: Final published version
ISSN
1064-8275
1095-7197