On invariance in hierarchical models
Author(s)
Bouvrie, Jacob Vincent; Rosasco, Lorenzo Andrea; Poggio, Tomaso A.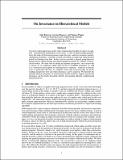
DownloadBouvrie-2014-On invariance.pdf (316.0Kb)
PUBLISHER_POLICY
Publisher Policy
Article is made available in accordance with the publisher's policy and may be subject to US copyright law. Please refer to the publisher's site for terms of use.
Terms of use
Metadata
Show full item recordAbstract
A goal of central importance in the study of hierarchical models for object recognition -- and indeed the visual cortex -- is that of understanding quantitatively the trade-off between invariance and selectivity, and how invariance and discrimination properties contribute towards providing an improved representation useful for learning from data. In this work we provide a general group-theoretic framework for characterizing and understanding invariance in a family of hierarchical models. We show that by taking an algebraic perspective, one can provide a concise set of conditions which must be met to establish invariance, as well as a constructive prescription for meeting those conditions. Analyses in specific cases of particular relevance to computer vision and text processing are given, yielding insight into how and when invariance can be achieved. We find that the minimal sets of transformations intrinsic to the hierarchical model needed to support a particular invariance can be clearly described, thereby encouraging efficient computational implementations.
Date issued
2009Department
Massachusetts Institute of Technology. Center for Biological & Computational Learning; Massachusetts Institute of Technology. Department of Brain and Cognitive Sciences; McGovern Institute for Brain Research at MITJournal
Advances in Neural Information Processing Systems (NIPS)
Publisher
Neural Information Processing Systems Foundation
Citation
Bouvrie, Jake, Lorenzo Rosasco, and Tomaso Poggio. "On invariance in Hierarchical models." Advances in Neural Information Processing 22 (NIPS 2009).
Version: Final published version
ISSN
1049-5258