Multitask Learning of Signaling and Regulatory Networks with Application to Studying Human Response to Flu
Author(s)
Jain, Siddhartha; Gitter, Anthony; Bar-Joseph, Ziv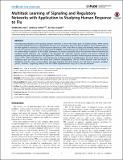
DownloadJain-2014-Multitask Learning o.pdf (1.257Mb)
PUBLISHER_CC
Publisher with Creative Commons License
Creative Commons Attribution
Terms of use
Metadata
Show full item recordAbstract
Reconstructing regulatory and signaling response networks is one of the major goals of systems biology. While several successful methods have been suggested for this task, some integrating large and diverse datasets, these methods have so far been applied to reconstruct a single response network at a time, even when studying and modeling related conditions. To improve network reconstruction we developed MT-SDREM, a multi-task learning method which jointly models networks for several related conditions. In MT-SDREM, parameters are jointly constrained across the networks while still allowing for condition-specific pathways and regulation. We formulate the multi-task learning problem and discuss methods for optimizing the joint target function. We applied MT-SDREM to reconstruct dynamic human response networks for three flu strains: H1N1, H5N1 and H3N2. Our multi-task learning method was able to identify known and novel factors and genes, improving upon prior methods that model each condition independently. The MT-SDREM networks were also better at identifying proteins whose removal affects viral load indicating that joint learning can still lead to accurate, condition-specific, networks. Supporting website with MT-SDREM implementation: http://sb.cs.cmu.edu/mtsdrem
Date issued
2014-12Department
Massachusetts Institute of Technology. Department of Biological EngineeringJournal
PLoS Computational Biology
Publisher
Public Library of Science
Citation
Jain, Siddhartha, Anthony Gitter, and Ziv Bar-Joseph. “Multitask Learning of Signaling and Regulatory Networks with Application to Studying Human Response to Flu.” Edited by Mona Singh. PLoS Comput Biol 10, no. 12 (December 18, 2014): e1003943.
Version: Final published version
ISSN
1553-7358
1553-734X