Inference on Counterfactual Distributions
Author(s)
Chernozhukov, Victor V.; Melly, Blaise; Fernandez-Val, Ivan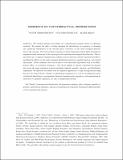
DownloadChernozhukov_Inference on.pdf (2.505Mb)
OPEN_ACCESS_POLICY
Open Access Policy
Creative Commons Attribution-Noncommercial-Share Alike
Terms of use
Metadata
Show full item recordAbstract
Counterfactual distributions are important ingredients for policy analysis and decomposition analysis in empirical economics. In this article, we develop modeling and inference tools for counterfactual distributions based on regression methods. The counterfactual scenarios that we consider consist of ceteris paribus changes in either the distribution of covariates related to the outcome of interest or the conditional distribution of the outcome given covariates. For either of these scenarios, we derive joint functional central limit theorems and bootstrap validity results for regression-based estimators of the status quo and counterfactual outcome distributions. These results allow us to construct simultaneous confidence sets for function-valued effects of the counterfactual changes, including the effects on the entire distribution and quantile functions of the outcome as well as on related functionals. These confidence sets can be used to test functional hypotheses such as no-effect, positive effect, or stochastic dominance. Our theory applies to general counterfactual changes and covers the main regression methods including classical, quantile, duration, and distribution regressions. We illustrate the results with an empirical application to wage decompositions using data for the United States.
As a part of developing the main results, we introduce distribution regression as a comprehensive and flexible tool for modeling and estimating the entire conditional distribution. We show that distribution regression encompasses the Cox duration regression and represents a useful alternative to quantile regression. We establish functional central limit theorems and bootstrap validity results for the empirical distribution regression process and various related functionals.
Date issued
2013-11Department
Massachusetts Institute of Technology. Department of EconomicsJournal
Econometrica
Publisher
The Econometric Society
Citation
Chernozhukov, Victor, Ivan Fernandez-Val and Blaise Melly. “Inference on Counterfactual Distributions.” Econometrica 81, no. 6 (2013): 2205–2268.
Version: Author's final manuscript
ISSN
0012-9682
1468-0262