Reactive point processes: A new approach to predicting power failures in underground electrical systems
Author(s)
Ertekin, Seyda; Rudin, Cynthia; McCormick, Tyler H.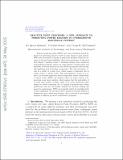
DownloadRudin_Reactive point.pdf (751.8Kb)
PUBLISHER_POLICY
Publisher Policy
Article is made available in accordance with the publisher's policy and may be subject to US copyright law. Please refer to the publisher's site for terms of use.
Terms of use
Metadata
Show full item recordAbstract
Reactive point processes (RPPs) are a new statistical model designed for predicting discrete events in time based on past history. RPPs were developed to handle an important problem within the domain of electrical grid reliability: short-term prediction of electrical grid failures (“manhole events”), including outages, fires, explosions and smoking manholes, which can cause threats to public safety and reliability of electrical service in cities. RPPs incorporate self-exciting, self-regulating and saturating components. The self-excitement occurs as a result of a past event, which causes a temporary rise in vulner ability to future events. The self-regulation occurs as a result of an external inspection which temporarily lowers vulnerability to future events. RPPs can saturate when too many events or inspections occur close together, which ensures that the probability of an event stays within a realistic range. Two of the operational challenges for power companies are (i) making continuous-time failure predictions, and (ii) cost/benefit analysis for decision making and proactive maintenance. RPPs are naturally suited for handling both of these challenges. We use the model to predict power-grid failures in Manhattan over a short-term horizon, and to provide a cost/benefit analysis of different proactive maintenance programs.
Date issued
2015-03Department
Sloan School of ManagementJournal
The Annals of Applied Statistics
Publisher
Institute of Mathematical Statistics
Citation
Ertekin, Seyda, Cynthia Rudin, and Tyler H. McCormick. “Reactive Point Processes: A New Approach to Predicting Power Failures in Underground Electrical Systems.” The Annals of Applied Statistics 9, no. 1 (March 2015): 122–144. © 2015 Institute of Mathematical Statistics
Version: Final published version
ISSN
1932-6157