Prediction of cartilage compressive modulus using multiexponential analysis of T[subscript 2] relaxation data and support vector regression
Author(s)
Irrechukwu, Onyi N.; Thaer, Sarah Von; Lin, Ping-Chang; Reiter, David A.; Grodzinsky, Alan J.; Spencer, Richard G.; Frank, Eliot; ... Show more Show less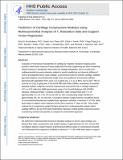
DownloadGrodzinsky_Prediction of.pdf (910.8Kb)
OPEN_ACCESS_POLICY
Open Access Policy
Creative Commons Attribution-Noncommercial-Share Alike
Terms of use
Metadata
Show full item recordAbstract
Evaluation of mechanical characteristics of cartilage by magnetic resonance imaging would provide a noninvasive measure of tissue quality both for tissue engineering and when monitoring clinical response to therapeutic interventions for cartilage degradation. We use results from multiexponential transverse relaxation analysis to predict equilibrium and dynamic stiffness of control and degraded bovine nasal cartilage, a biochemical model for articular cartilage. Sulfated glycosaminoglycan concentration/wet weight (ww) and equilibrium and dynamic stiffness decreased with degradation from 103.6 ± 37.0 µg/mg ww, 1.71 ± 1.10 MPa and 15.3 ± 6.7 MPa in controls to 8.25 ± 2.4 µg/mg ww, 0.015 ± 0.006 MPa and 0.89 ± 0.25MPa, respectively, in severely degraded explants. Magnetic resonance measurements were performed on cartilage explants at 4 °C in a 9.4 T wide-bore NMR spectrometer using a Carr–Purcell–Meiboom–Gill sequence. Multiexponential T[subscript 2] analysis revealed four water compartments with T[subscript 2] values of approximately 0.14, 3, 40 and 150 ms, with corresponding weight fractions of approximately 3, 2, 4 and 91%. Correlations between weight fractions and stiffness based on conventional univariate and multiple linear regressions exhibited a maximum r[superscript 2] of 0.65, while those based on support vector regression (SVR) had a maximum r[superscript 2] value of 0.90. These results indicate that (i) compartment weight fractions derived from multiexponential analysis reflect cartilage stiffness and (ii) SVR-based multivariate regression exhibits greatly improved accuracy in predicting mechanical properties as compared with conventional regression.
Date issued
2014-02Department
Massachusetts Institute of Technology. Department of Biological Engineering; Massachusetts Institute of Technology. Department of Mechanical EngineeringJournal
NMR in Biomedicine
Publisher
Wiley Blackwell
Citation
Irrechukwu, Onyi N., Sarah Von Thaer, Eliot H. Frank, Ping-Chang Lin, David A. Reiter, Alan J. Grodzinsky, and Richard G. Spencer. “Prediction of Cartilage Compressive Modulus Using Multiexponential Analysis of T[subscript 2] Relaxation Data and Support Vector Regression.” NMR Biomed. 27, no. 4 (February 12, 2014): 468–477.
Version: Author's final manuscript
ISSN
09523480
1099-1492