Identification of signaling pathways related to drug efficacy in hepatocellular carcinoma via integration of phosphoproteomic, genomic and clinical data
Author(s)
Melas, Ioannis N.; Lauffenburger, Douglas A.; Alexopoulos, Leonidas G.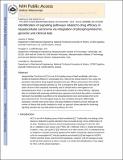
DownloadLauffenburger_Identification of.pdf (629.8Kb)
OPEN_ACCESS_POLICY
Open Access Policy
Creative Commons Attribution-Noncommercial-Share Alike
Terms of use
Metadata
Show full item recordAbstract
Hepatocellular Carcinoma (HCC) is one of the leading causes of death worldwide, with only a handful of treatments effective in unresectable HCC. Most of the clinical trials for HCC using new generation interventions (drug-targeted therapies) have poor efficacy whereas just a few of them show some promising clinical outcomes [1]. This is amongst the first studies where the mode of action of some of the compounds extensively used in clinical trials is interrogated on the phosphoproteomic level, in an attempt to build predictive models for clinical efficacy. Signaling data are combined with previously published gene expression and clinical data within a consistent framework that identifies drug effects on the phosphoproteomic level and translates them to the gene expression level. The interrogated drugs are then correlated with genes differentially expressed in normal versus tumor tissue, and genes predictive of patient survival. Although the number of clinical trial results considered is small, our approach shows potential for discerning signaling activities that may help predict drug efficacy for HCC.
Date issued
2013-11Department
Massachusetts Institute of Technology. Department of Biological EngineeringJournal
Proceedings of the 13th IEEE International Conference on BioInformatics and BioEngineering
Publisher
Institute of Electrical and Electronics Engineers (IEEE)
Citation
Melas, Ioannis N., Douglas A. Lauffenburger, and Leonidas G. Alexopoulos. “Identification of Signaling Pathways Related to Drug Efficacy in Hepatocellular Carcinoma via Integration of Phosphoproteomic, Genomic and Clinical Data.” 13th IEEE International Conference on BioInformatics and BioEngineering (November 2013).
Version: Author's final manuscript
ISBN
978-1-4799-3163-7