Near-Lossless Compression for Large Traffic Networks
Author(s)
Asif, Muhammad Tayyab; Srinivasan, Kannan; Mitrovic, Nikola; Dauwels, Justin; Jaillet, Patrick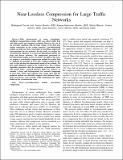
DownloadJaillet_Near-lossless.pdf (1.217Mb)
OPEN_ACCESS_POLICY
Open Access Policy
Creative Commons Attribution-Noncommercial-Share Alike
Terms of use
Metadata
Show full item recordAbstract
With advancements in sensor technologies, intelligent transportation systems can collect traffic data with high spatial and temporal resolution. However, the size of the networks combined with the huge volume of the data puts serious constraints on system resources. Low-dimensional models can help ease these constraints by providing compressed representations for the networks. In this paper, we analyze the reconstruction efficiency of several low-dimensional models for large and diverse networks. The compression performed by low-dimensional models is lossy in nature. To address this issue, we propose a near-lossless compression method for traffic data by applying the principle of lossy plus residual coding. To this end, we first develop a low-dimensional model of the network. We then apply Huffman coding (HC) in the residual layer. The resultant algorithm guarantees that the maximum reconstruction error will remain below a desired tolerance limit. For analysis, we consider a large and heterogeneous test network comprising of more than 18 000 road segments. The results show that the proposed method can efficiently compress data obtained from a large and diverse road network, while maintaining the upper bound on the reconstruction error.
Date issued
2015-07Department
Massachusetts Institute of Technology. Department of Electrical Engineering and Computer Science; Massachusetts Institute of Technology. Operations Research CenterJournal
IEEE Transactions on Intelligent Transportation Systems
Publisher
Institute of Electrical and Electronics Engineers (IEEE)
Citation
Asif, Muhammad Tayyab, Kannan Srinivasan, Nikola Mitrovic, Justin Dauwels, and Patrick Jaillet. “Near-Lossless Compression for Large Traffic Networks.” IEEE Transactions on Intelligent Transportation Systems 16, no. 4 (August 2015): 1817–1826.
Version: Author's final manuscript
ISSN
1524-9050
1558-0016