Entropy-Scaling Search of Massive Biological Data
Author(s)
Yu, Yun William; Daniels, Noah; Danko, David C.; Berger Leighton, Bonnie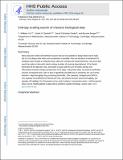
DownloadBerger_Entropy-scaling.pdf (751.3Kb)
PUBLISHER_CC
Publisher with Creative Commons License
Creative Commons Attribution
Terms of use
Metadata
Show full item recordAbstract
Many datasets exhibit a well-defined structure that can be exploited to design faster search tools, but it is not always clear when such acceleration is possible. Here, we introduce a framework for similarity search based on characterizing a dataset’s entropy and fractal dimension. We prove that searching scales in time with metric entropy (number of covering hyperspheres), if the fractal dimension of the dataset is low, and scales in space with the sum of metric entropy and information-theoretic entropy (randomness of the data). Using these ideas, we present accelerated versions of standard tools, with no loss in specificity and little loss in sensitivity, for use in three domains—high-throughput drug screening (Ammolite, 150× speedup), metagenomics (MICA, 3.5× speedup of DIAMOND [3,700× BLASTX]), and protein structure search (esFragBag, 10× speedup of FragBag). Our framework can be used to achieve “‘compressive omics,” and the general theory can be readily applied to data science problems outside of biology (source code: http://gems.csail.mit.edu).
Date issued
2015-08Department
Massachusetts Institute of Technology. Computer Science and Artificial Intelligence Laboratory; Massachusetts Institute of Technology. Department of MathematicsJournal
Cell Systems
Publisher
Elsevier
Citation
Yu, Y. William, Noah M. Daniels, David Christian Danko, and Bonnie Berger. “Entropy-Scaling Search of Massive Biological Data.” Cell Systems 1, no. 2 (August 2015): 130–140.
Version: Author's final manuscript
ISSN
24054712