A convex approach to blind deconvolution with diverse inputs
Author(s)
Ahmed, Ali; Cosse, Augustin M.; Demanet, Laurent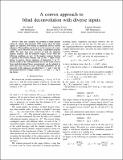
DownloadDemanet_A convex.pdf (324.4Kb)
OPEN_ACCESS_POLICY
Open Access Policy
Creative Commons Attribution-Noncommercial-Share Alike
Terms of use
Metadata
Show full item recordAbstract
This note considers the problem of blind identification of a linear, time-invariant (LTI) system when the input signals are unknown, but belong to sufficiently diverse, known subspaces. This problem can be recast as the recovery of a rank-1 matrix, and is effectively relaxed using a semidefinite program (SDP). We show that exact recovery of both the unknown impulse response, and the unknown inputs, occurs when the following conditions are met: (1) the impulse response function is spread in the Fourier domain, and (2) the N input vectors belong to generic, known subspaces of dimension K in ℝL. Recent results in the well-understood area of low-rank recovery from underdetermined linear measurements can be adapted to show that exact recovery occurs with high probablility (on the genericity of the subspaces) provided that K,L, and N obey the information-theoretic scalings, namely L ≳ K and N ≳ 1 up to log factors.
Date issued
2015-12Department
Massachusetts Institute of Technology. Department of MathematicsJournal
2015 IEEE 6th International Workshop on Computational Advances in Multi-Sensor Adaptive Processing (CAMSAP)
Publisher
Institute of Electrical and Electronics Engineers (IEEE)
Citation
Ahmed, Ali, Augustin Cosse, and Laurent Demanet. “A Convex Approach to Blind Deconvolution with Diverse Inputs.” 2015 IEEE 6th International Workshop on Computational Advances in Multi-Sensor Adaptive Processing (CAMSAP) (December 2015).
Version: Author's final manuscript
ISBN
978-1-4799-1963-5