Estimating the parameters of system dynamics models using indirect inference
Author(s)
Hosseinichimeh, Niyousha; Wittenborn, Andrea K.; Rahmandad, Hazhir; Jalali, Seyed Mohammad Javad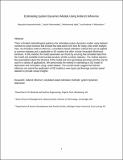
DownloadHosseiniChimeh-Rahmandad-Etal2017-Indirect Inference ArticleFinal.pdf (1.192Mb)
OPEN_ACCESS_POLICY
Open Access Policy
Creative Commons Attribution-Noncommercial-Share Alike
Terms of use
Metadata
Show full item recordAbstract
There is limited methodological guidance for estimating system dynamics (SD) models using datasets common to social sciences that include few data points over time for many units under analysis. Here, we introduce indirect inference, a simulation-based estimation method that can be applied to common datasets and is applicable to SD models that often include intractable likelihood functions. In this method, the model parameters are found by ensuring that simulated data from the model and available empirical data produce similar auxiliary statistics. The method requires few assumptions about the structure of the model and error-generating processes and thus can be used in a variety of applications. We demonstrate the method in estimating an SD model of depression and rumination using a panel dataset. The overall results suggest that indirect inference can extend the application of SD models to new topics and leverage common panel datasets to provide unique insights.
Date issued
2017-01Department
Sloan School of ManagementJournal
System Dynamics Review
Publisher
Wiley Blackwell
Citation
Hosseinichimeh, Niyousha et al. “Estimating the Parameters of System Dynamics Models Using Indirect Inference.” System Dynamics Review 32, 2 (April 2016): 156–180 © 2016 System Dynamics Society
Version: Author's final manuscript
ISSN
0883-7066
1099-1727