Learning a probabilistic latent space of object shapes via 3D generative-adversarial modeling
Author(s)
Wu, Jiajun; Zhang, Chengkai; Xue, Tianfan; Freeman, William T.; Tenenbaum, Joshua B.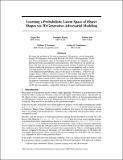
Download6096-learning-a-probabilistic-latent-space-of-object-shapes-via-3d-generative-adversarial-modeling.pdf (7.688Mb)
PUBLISHER_POLICY
Publisher Policy
Article is made available in accordance with the publisher's policy and may be subject to US copyright law. Please refer to the publisher's site for terms of use.
Terms of use
Metadata
Show full item recordAbstract
We study the problem of 3D object generation. We propose a novel framework, namely 3D Generative Adversarial Network (3D-GAN), which generates 3D objects from a probabilistic space by leveraging recent advances in volumetric convo-lutional networks and generative adversarial nets. The benefits of our model are three-fold: first, the use of an adversarial criterion, instead of traditional heuristic criteria, enables the generator to capture object structure implicitly and to synthesize high-quality 3D objects; second, the generator establishes a mapping from a low-dimensional probabilistic space to the space of 3D objects, so that we can sample objects without a reference image or CAD models, and explore the 3D object manifold; third, the adversarial discriminator provides a powerful 3D shape descriptor which, learned without supervision, has wide applications in 3D object recognition. Experiments demonstrate that our method generates high-quality 3D objects, and our unsupervisedly learned features achieve impressive performance on 3D object recognition, comparable with those of supervised learning methods.
Date issued
2016-12Department
Massachusetts Institute of Technology. Computer Science and Artificial Intelligence Laboratory; Massachusetts Institute of Technology. Department of Electrical Engineering and Computer ScienceJournal
Advances in Neural Information Processing Systems 29 (NIPS 2016)
Publisher
Neural Information Processing Systems Foundation
Citation
Wu, Jiajun et al. "Learning a Probabilistic Latent Space of Object Shapes via 3D Generative-Adversarial Modeling." Advances in Neural Information Processing Systems 29 (NIPS 2016), Barcelona, Spain, December 5-10, 2016. © 2016 Neural Information Processing Systems Foundation
Version: Final published version