Robustly Learning a Gaussian: Getting Optimal Error, Efficiently
Author(s)
Stewart, Alistair; Diakonikolas, Ilias; Kamath, Gautam Chetan; Kane, Daniel M; Li, Jerry Zheng; Moitra, Ankur; ... Show more Show less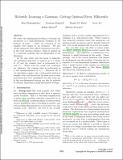
Download1.9781611975031.171.pdf (539.0Kb)
PUBLISHER_POLICY
Publisher Policy
Article is made available in accordance with the publisher's policy and may be subject to US copyright law. Please refer to the publisher's site for terms of use.
Terms of use
Metadata
Show full item recordAbstract
We study the fundamental problem of learning the parameters of a high-dimensional Gaussian in the presence of noise | where an "-fraction of our samples were chosen by an adversary. We give robust estimators that achieve estimation error O(ϵ) in the total variation distance, which is optimal up to a universal constant that is independent of the dimension. In the case where just the mean is unknown, our robustness guarantee is optimal up to a factor of p 2 and the running time is polynomial in d and 1/ϵ. When both the mean and covariance are unknown, the running time is polynomial in d and quasipolynomial in 1/ϵ. Moreover all of our algorithms require only a polynomial number of samples. Our work shows that the same sorts of error guarantees that were established over fifty years ago in the one-dimensional setting can also be achieved by efficient algorithms in high-dimensional settings.
Date issued
2018-01Department
Massachusetts Institute of Technology. Computer Science and Artificial Intelligence Laboratory; Massachusetts Institute of Technology. Department of Electrical Engineering and Computer Science; Massachusetts Institute of Technology. Department of Mathematics; Massachusetts Institute of Technology. Department of PhysicsJournal
Proceedings of the Twenty-Ninth Annual ACM-SIAM Symposium on Discrete Algorithms
Publisher
Society for Industrial and Applied Mathematics
Citation
Diakonikolas, Ilias, Gautam Kamath, Daniel M. Kane, Jerry Li, Ankur Moitra, and Alistair Stewart. “Robustly Learning a Gaussian: Getting Optimal Error, Efficiently.” Proceedings of the Twenty-Ninth Annual ACM-SIAM Symposium on Discrete Algorithms (January 2018): 2683–2702.
Version: Final published version
ISSN
0368-4245