Inference and Evolutionary Analysis of Genome-Scale Regulatory Networks in Large Phylogenies
Author(s)
Koch, Christopher; Konieczka, Jay; Delorey, Toni; Socha, Amanda; Davis, Kathleen; Knaack, Sara A.; Thompson, Dawn; O'Shea, Erin K.; Regev, Aviv; Roy, Sushmita; Lyons, Ana M.; ... Show more Show less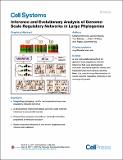
Download1-s2.0-S2405471217301783-main.pdf (5.336Mb)
PUBLISHER_CC
Publisher with Creative Commons License
Creative Commons Attribution
Terms of use
Metadata
Show full item recordAbstract
Changes in transcriptional regulatory networks can significantly contribute to species evolution and adaptation. However, identification of genome-scale regulatory networks is an open challenge, especially in non-model organisms. Here, we introduce multi-species regulatory network learning (MRTLE), a computational approach that uses phylogenetic structure, sequence-specific motifs, and transcriptomic data, to infer the regulatory networks in different species. Using simulated data from known networks and transcriptomic data from six divergent yeasts, we demonstrate that MRTLE predicts networks with greater accuracy than existing methods because it incorporates phylogenetic information. We used MRTLE to infer the structure of the transcriptional networks that control the osmotic stress responses of divergent, non-model yeast species and then validated our predictions experimentally. Interrogating these networks reveals that gene duplication promotes network divergence across evolution. Taken together, our approach facilitates study of regulatory network evolutionary dynamics across multiple poorly studied species. Keywords: regulatory networks;
network inference; evolution of gene regulatory networks; evolution of stress response; yeast; probabilistic graphical model; phylogeny; comparative functional genomics
Date issued
2017-05Department
Massachusetts Institute of Technology. Department of BiologyJournal
Cell Systems
Publisher
Elsevier
Citation
Koch, Christopher et al. “Inference and Evolutionary Analysis of Genome-Scale Regulatory Networks in Large Phylogenies.” Cell Systems 4, 5 (May 2017): 543–558 © 2017 The Author(s)
Version: Final published version
ISSN
2405-4712