Multifidelity Uncertainty Propagation via Adaptive Surrogates in Coupled Multidisciplinary Systems
Author(s)
Chaudhuri, Anirban; Lam, Remi; Willcox, Karen E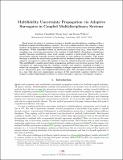
DownloadMultifidelity-uncertainty-quantification-Chaudhuri-Lam-Willcox.pdf (3.581Mb)
OPEN_ACCESS_POLICY
Open Access Policy
Creative Commons Attribution-Noncommercial-Share Alike
Terms of use
Metadata
Show full item recordAbstract
Fixed point iteration is a common strategy to handle interdisciplinary coupling within a feedback-coupled multidisciplinary analysis. For each coupled analysis, this requires a large number of disciplinary high-fidelity simulations to resolve the interactions between different disciplines. When embedded within an uncertainty analysis loop (e.g., with Monte Carlo sampling over uncertain parameters), the number of high-fidelity disciplinary simulations quickly becomes prohibitive, because each sample requires a fixed point iteration and the uncertainty analysis typically involves thousands or even millions of samples. This paper develops a method for uncertainty quantification in feedback-coupled systems that leverage adaptive surrogates to reduce the number of cases forwhichfixedpoint iteration is needed. The multifidelity coupled uncertainty propagation method is an iterative process that uses surrogates for approximating the coupling variables and adaptive sampling strategies to refine the surrogates. The adaptive sampling strategies explored in this work are residual error, information gain, and weighted information gain. The surrogate models are adapted in a way that does not compromise the accuracy of the uncertainty analysis relative to the original coupled high-fidelity problem as shown through a rigorous convergence analysis.
Date issued
2017-08Department
Massachusetts Institute of Technology. Department of Aeronautics and AstronauticsJournal
AIAA Journal
Publisher
American Institute of Aeronautics and Astronautics (AIAA)
Citation
Chaudhuri, Anirban, et al. “Multifidelity Uncertainty Propagation via Adaptive Surrogates in Coupled Multidisciplinary Systems.” AIAA Journal, vol. 56, no. 1, Jan. 2018, pp. 235–49. © 2017 by Anirban Chaudhuri, Remi Lam, and Karen Willcox
Version: Author's final manuscript
ISSN
0001-1452
1533-385X