Visualizing a neural network that develops quantum perturbation theory
Author(s)
Wu, Yadong; Zhang, Pengfei; Shen, Huitao; Zhai, Hui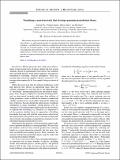
DownloadPhysRevA.98.010701.pdf (904.5Kb)
PUBLISHER_POLICY
Publisher Policy
Article is made available in accordance with the publisher's policy and may be subject to US copyright law. Please refer to the publisher's site for terms of use.
Terms of use
Metadata
Show full item recordAbstract
Motivated by the question whether the empirical fitting of data by neural networks can yield the same structure of physical laws, we apply neural networks to a quantum-mechanical two-body scattering problem with short-range potentials—a problem that by itself plays an important role in many branches of physics. After training, the neural network can accurately predict s-wave scattering length, which governs the low-energy scattering physics. By visualizing the neural network, we show that it develops perturbation theory order by order when the potential depth increases, without solving the Schrödinger equation or obtaining the wave function explicitly. The result provides an important benchmark to the machine-assisted physics research or even automated machine learning physics laws.
Date issued
2018-07Department
Massachusetts Institute of Technology. Department of PhysicsJournal
Physical Review A
Publisher
American Physical Society
Citation
Wu, Yadong, Pengfei Zhang, Huitao Shen and Hui Zhai. "Visualizing a neural network that develops quantum perturbation theory." Physucial Review A 98 (2018), 010701.
Version: Final published version
ISSN
2469-9926
2469-9934