Deep learning to predict the lab-of-origin of engineered DNA
Author(s)
Nielsen, Alec Andrew; Voigt, Christopher A.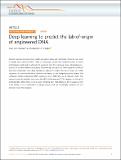
Downloads41467-018-05378-z.pdf (998.7Kb)
PUBLISHER_CC
Publisher with Creative Commons License
Creative Commons Attribution
Terms of use
Metadata
Show full item recordAbstract
Genetic engineering projects are rapidly growing in scale and complexity, driven by new tools to design and construct DNA. There is increasing concern that widened access to these technologies could lead to attempts to construct cells for malicious intent, illegal drug production, or to steal intellectual property. Determining the origin of a DNA sequence is difficult and time-consuming. Here deep learning is applied to predict the lab-of-origin of a DNA sequence. A convolutional neural network was trained on the Addgene plasmid dataset that contained 42,364 engineered DNA sequences from 2230 labs as of February 2016. The network correctly identifies the source lab 48% of the time and 70% it appears in the top 10 predicted labs. Often, there is not a single “smoking gun” that affiliates a DNA sequence with a lab. Rather, it is a combination of design choices that are individually common but collectively reveal the designer.
Date issued
2018-08Department
Massachusetts Institute of Technology. Department of Biological Engineering; Massachusetts Institute of Technology. Synthetic Biology CenterJournal
Nature Communications
Publisher
Nature Publishing Group
Citation
Nielsen, Alec A. K., and Christopher A. Voigt. “Deep Learning to Predict the Lab-of-Origin of Engineered DNA.” Nature Communications 9, 1 (August 2018): 3135 © 2018 The Author(s)
Version: Final published version
ISSN
2041-1723