Reliably Arranging Objects in Uncertain Domains
Author(s)
Anders, Ariel S; Kaelbling, Leslie P; Lozano-Perez, Tomas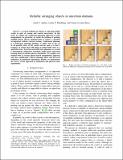
DownloadAccepted version (3.425Mb)
Terms of use
Metadata
Show full item recordAbstract
A crucial challenge in robotics is achieving reliable results in spite of sensing and control uncertainty. In this work, we explore the conformant planning approach to robot manipulation. In particular, we tackle the problem of pushing multiple planar objects simultaneously to achieve a specified arrangement without external sensing. Conformant planning is a belief-state planning problem. A belief state is the set of all possible states of the world, and the goal is to find a sequence of actions that will bring an initial belief state to a goal belief state. To do forward belief-state planning, we created a deterministic belief-state transition model from supervised learning based on off-line physics simulations. We compare our method with an on-line physics-based manipulation approach and show significantly reduced planning times and increased robustness in simulated experiments. Finally, we demonstrate the success of this approach in simulations and physical robot experiments.
Date issued
2018-09-28Department
Massachusetts Institute of Technology. Department of Electrical Engineering and Computer ScienceJournal
2018 IEEE International Conference on Robotics and Automation (ICRA)
Publisher
Institute of Electrical and Electronics Engineers (IEEE)
Citation
Anders, Ariel S., et al. “Reliably Arranging Objects in Uncertain Domains.” 2018 IEEE International Conference on Robotics and Automation (ICRA), 21-25 May, 2018, Brisbane, Queensland, Australia, IEEE, 2018, pp. 1603–10.
Version: Author's final manuscript
ISSN
2577-087X