Nonconvex Generalized Benders Decomposition for Stochastic Separable Mixed-Integer Nonlinear Programs
Author(s)
Li, Xiang; Tomasgard, Asgeir; Barton, Paul I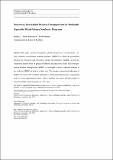
DownloadAccepted version (337.8Kb)
Terms of use
Metadata
Show full item recordAbstract
This paper considers deterministic global optimization of scenario-based, two-stage stochastic mixed-integer nonlinear programs (MINLPs) in which the participating functions are nonconvex and separable in integer and continuous variables. A novel decomposition method based on generalized Benders decomposition, named nonconvex generalized Benders decomposition (NGBD), is developed to obtain ε-optimal solutions of the stochastic MINLPs of interest in finite time. The dramatic computational advantage of NGBD over state-of-the-art global optimizers is demonstrated through the computational study of several engineering problems, where a problem with almost 150,000 variables is solved by NGBD within 80 minutes of solver time. Keywords: stochastic programming; mixed-integer nonlinear programming; decomposition algorithm; global optimization
Date issued
2011-07Department
Massachusetts Institute of Technology. Process Systems Engineering Laboratory; Massachusetts Institute of Technology. Department of Chemical EngineeringJournal
Journal of Optimization Theory and Applications
Publisher
Springer Science and Business Media LLC
Citation
Li, Xiang et al. "Nonconvex generalized benders decomposition for stochastic separable mixed-integer nonlinear programs." Journal of Optimization Theory and Applications 151 (December 2011): 425 © 2011 Publisher
Version: Author's final manuscript
ISSN
0022-3239
1573-2878