Deep Inference for Covariance Estimation: Learning Gaussian Noise Models for State Estimation
Author(s)
Liu, Katherine; Ok, Kyel; Vega-Brown, William R; Roy, Nicholas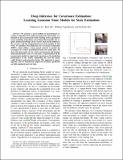
Downloadliu_ok_icra18.pdf (1.191Mb)
Terms of use
Metadata
Show full item recordAbstract
We present a novel method of measurement covariance estimation that models measurement uncertainty as a function of the measurement itself. Existing work in predictive sensor modeling outperforms conventional fixed models, but requires domain knowledge of the sensors that heavily influences the accuracy and the computational cost of the models. In this work, we introduce Deep Inference for Covariance Estimation (DICE), which utilizes a deep neural network to predict the covariance of a sensor measurement from raw sensor data. We show that given pairs of raw sensor measurement and ground-truth measurement error, we can learn a representation of the measurement model via supervised regression on the prediction performance of the model, eliminating the need for hand-coded features and parametric forms. Our approach is sensor-agnostic, and we demonstrate improved covariance prediction on both simulated and real data. Keywords: robot sensing systems; measurement uncertainty; measurement errors; covariance matrices; predictive models; estimation; neural networks
Date issued
2018-09-13Department
Massachusetts Institute of Technology. Computer Science and Artificial Intelligence LaboratoryJournal
2018 IEEE International Conference on Robotics and Automation (ICRA)
Publisher
IEEE
Citation
Liu, Katherine et al. "Deep Inference for Covariance Estimation: Learning Gaussian Noise Models for State Estimation." 2018 IEEE International Conference on Robotics and Automation (ICRA), May 21-25, 2018, Brisbane, Queensland, Australia, IEEE, 2018
Version: Author's final manuscript
ISBN
9781538630815
9781538630808
9781538630822
ISSN
2577-087X